The Ethics of Artificial Intelligence
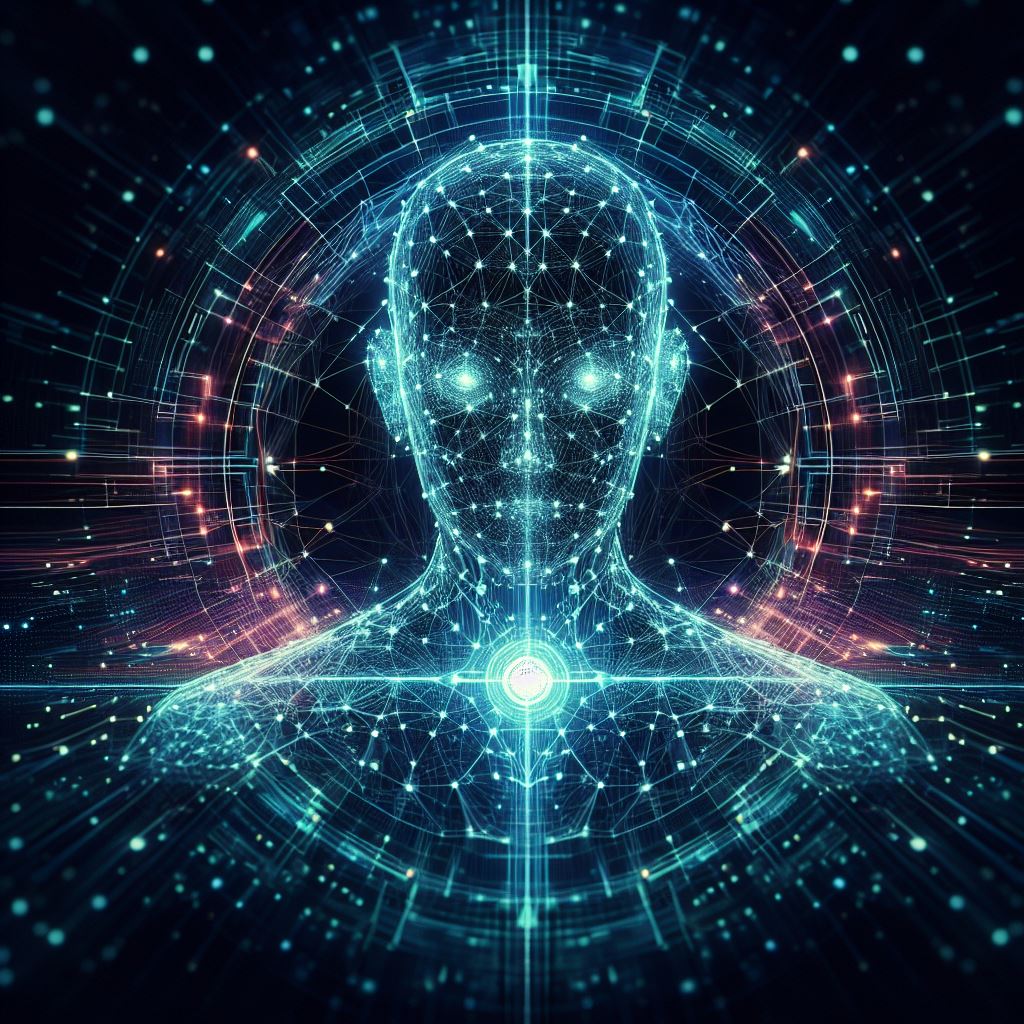
The ethics of artificial intelligence (AI) lie at the intersection of technology and humanity, demanding careful consideration of moral implications. As AI systems become increasingly integrated into our daily lives, questions surrounding fairness, transparency, accountability, and privacy emerge as critical concerns. Ensuring that AI is developed and deployed in a manner that upholds human rights and promotes societal well-being is imperative. Ethical frameworks must address issues such as algorithmic bias, the impact on employment, autonomous decision-making, and the potential for misuse. Striking a balance between innovation and responsibility is paramount to harnessing the transformative potential of AI while safeguarding against unintended consequences. Collaboration between technologists, policymakers, ethicists, and the broader community is essential to navigating the complex ethical landscape of artificial intelligence.
What are the 3 main concerns about the ethics of AI
Here are 3 major ethical concerns surrounding AI;
- Bias: AI systems can inherit and amplify biases that exist in the data they’re trained on. This can lead to discriminatory outcomes, for example, in loan approvals or job applications. Imagine an AI analyzing loan applications and finding a pattern of men getting approved more than women. The AI might decide this is the norm and continue to favor men.
- Transparency: Understanding the decision-making processes of numerous AI systems is challenging due to their complexity and lack of transparency. This lack of transparency can make it hard to hold developers or users accountable for biased or unfair outcomes. You might not know why an AI denied your loan application and have no way to challenge it.
- Privacy: AI development often relies on vast amounts of personal data. Collection and use of this data raises privacy concerns, especially if it’s used without people’s knowledge or consent. Imagine a company using your social media data to decide your insurance rates without you knowing.
What are the key ethical issues in AI
Several key ethical issues in artificial intelligence (AI) include:
- Bias and Fairness: AI systems can perpetuate and even exacerbate biases present in their training data, leading to discriminatory outcomes in decision-making processes such as hiring, lending, and criminal justice.
- Transparency and Accountability: The opacity of AI algorithms and decision-making processes raises concerns about accountability, making it difficult to understand how decisions are reached and to whom responsibility should be assigned in case of errors or harm.
- Privacy: AI systems often rely on vast amounts of personal data, raising significant privacy concerns regarding the collection, storage, and use of this data, particularly when it comes to sensitive information.
- Autonomy and Responsibility: As AI systems become more autonomous, questions arise about where responsibility lies for their actions and decisions, especially in cases where AI systems operate independently of human intervention.
- Employment and Socioeconomic Impact: The widespread adoption of AI technologies has the potential to disrupt labor markets, leading to job displacement and exacerbating socioeconomic inequalities.
- Security and Safety: AI systems can be vulnerable to adversarial attacks and manipulation, posing risks to cybersecurity and public safety, especially in critical domains such as autonomous vehicles, healthcare, and infrastructure.
- Ethical Governance and Regulation: There is a pressing need for robust ethical guidelines, regulations, and oversight mechanisms to ensure that AI development and deployment align with societal values and priorities, and to mitigate potential harms.
Addressing these ethical issues requires interdisciplinary collaboration among technologists, policymakers, ethicists, and other stakeholders to develop frameworks that prioritize human well-being, fairness, and accountability in the design, development, and deployment of AI systems.
What are the pillars of AI ethics
The pillars of AI ethics are the core principles that guide the development and use of AI in a responsible and trustworthy way. There can be some variation depending on the specific framework, but some of the key pillars include;
- Fairness: This ensures that AI systems are unbiased and don’t discriminate against certain groups. It involves looking at the data used to train AI and mitigating any biases that might be present.
- Transparency: This means understanding how AI systems arrive at their decisions. This allows for auditing and debugging of AI models to ensure they are functioning correctly.
- Accountability: There should be clear ownership and responsibility for the development, deployment, and outcomes of AI systems. This is especially important for high-stakes decisions made by AI.
- Privacy: AI development and use should respect individual privacy rights. This means collecting data ethically, using it for its intended purpose, and having strong security measures in place.
- Security: AI systems themselves should be secure from hacking or manipulation to prevent malicious use.
- Safety: AI systems should be designed and used in a way that minimizes risks of harm to people and the environment.
- Human well-being: AI should be used to benefit humanity and improve people’s lives. This means considering the social and ethical implications of AI development and deployment.
Can AI be biased
Yes, AI can be biased. AI systems are susceptible to bias in several ways;
- Biased data: AI systems learn from the data they’re trained on. If that data contains inherent biases, the AI will inherit those biases as well. For example, an AI trained on loan applications that were historically more likely to approve men than women might perpetuate that bias in its own decisions.
- Algorithmic bias: The algorithms used to develop AI can also introduce bias. Certain algorithms might favor certain patterns or features in the data, which could lead to biased outcomes.
- Human bias: The developers and programmers who create AI systems can also introduce bias, either intentionally or unintentionally. Their assumptions and perspectives can influence the way they design and train the AI.
Here are some real-world examples of AI bias;
- Facial recognition software: Some facial recognition systems are less accurate in recognizing people of color. This could have serious consequences, such as leading to wrongful arrests.
- Loan approvals: AI algorithms used in loan approvals might disadvantage certain groups based on factors like zip code or name.
- Hiring algorithms: AI used in resume screening could favor candidates with resumes that contain certain keywords, even if those keywords aren’t necessarily indicative of job performance. This could lead to qualified candidates being overlooked.
AI bias is a serious concern because it can lead to discrimination and unfair outcomes. Researchers and developers are working on ways to mitigate bias in AI, but it’s an ongoing challenge.
What are the 7 principles of trustworthy AI
The concept of trustworthy AI goes beyond just ethics and encompasses a broader set of principles. Here are the 7 key requirements according to the European Commission’s AI Ethics Guidelines for Trustworthy AI:
- Human agency and oversight: This principle emphasizes that AI should empower humans and not replace them entirely. There should be mechanisms for human oversight and control over AI systems, particularly for critical decisions.
- Technical robustness and safety: AI systems should be designed and built to be reliable, accurate, and secure. This includes having safeguards in place to prevent malfunctions and mitigate potential risks.
- Privacy and data governance: This principle ensures that AI development and use respect individual privacy rights. It involves collecting data ethically, using it for its intended purpose, and having strong security measures to protect personal information.
- Transparency: There should be transparency in how AI systems work and how they arrive at decisions. This allows for understanding, auditing, and debugging of AI models to ensure they are functioning correctly and without bias.
- Diversity, non-discrimination, and fairness: AI systems should be fair and avoid discrimination against any particular group. This requires careful consideration of the data used to train AI and taking steps to mitigate bias.
- Environmental and societal well-being: The development and deployment of AI should consider its impact on society and the environment. AI should be used for positive social good and to minimize potential harm.
- Accountability: Accountability should be clearly established for all stages of AI system development, implementation, and the resulting impacts. This is especially important for high-stakes decisions made by AI.
How do AI algorithms impact fairness and bias
AI algorithms can have a significant impact on fairness and bias, in both positive and negative ways. Here’s a breakdown of the key issues;
How AI can perpetuate bias
- Biased training data: AI systems, particularly machine learning, are trained on massive datasets. If this data contains inherent biases, the algorithms will learn and amplify those biases. For example, an algorithm trained on loan applications that were historically denied to women might perpetuate gender bias in future lending decisions.
- Algorithmic bias: Even unbiased data can lead to biased algorithms. The way algorithms are designed can introduce bias, such as giving more weight to certain factors that unfairly disadvantage certain groups.
Real-world examples of bias in AI:
- Criminal justice: Algorithms used to assess the risk of recidivism have been shown to disproportionately label people of color as high-risk.
- Facial recognition: Facial recognition software can have lower accuracy rates for people of color, leading to potential misuse in surveillance or law enforcement.
Positive potential of AI for fairness
- Mitigating human bias: AI can potentially reduce bias in human decision-making by automating tasks where human biases might creep in.
- Identifying bias: AI tools can be used to analyze data and identify potential biases in existing systems.
What are the risks of AI in terms of privacy
AI poses several significant risks to our privacy. Here’s a breakdown of the main concerns;
- Data Collection and Surveillance: AI systems are data hungry, and their effectiveness hinges on vast amounts of personal information. This can lead to excessive data collection practices, often exceeding what’s necessary for the stated purpose.
- Invasive Surveillance: AI can be used to analyze data from various sources, like cameras, social media, and browsing history, creating a detailed profile of individuals. This raises concerns about intrusive surveillance that could erode our sense of privacy and freedom.
- Data Breaches and Misuse: With so much personal data concentrated in AI systems, the risk of data breaches is significant. If such breaches occur, malicious actors could exploit sensitive information for identity theft, cyberbullying, or other harmful purposes.
- Predictive Harm: AI can infer highly personal details from seemingly unrelated data. This “predictive harm” could expose sensitive information like health conditions, political views, or sexual orientation, even if not directly collected.
- Lack of Transparency and Control: The inner workings of many AI systems are complex and opaque. This lack of transparency makes it difficult for individuals to understand how their data is being used and to exercise control over it.
- Algorithmic Bias: AI systems trained on biased data can perpetuate or amplify those biases in real-world applications. This could lead to discriminatory outcomes in areas like loan approvals, job hiring, or law enforcement.
Here are some things being done to address these privacy risks;
- Data privacy regulations: Many countries are developing and implementing stricter data privacy regulations to give individuals more control over their information.
- Focus on data minimization: There’s a growing emphasis on collecting only the data essential for AI systems to function and anonymizing data whenever possible.
- Explainable AI: Researchers are developing techniques to make AI algorithms more transparent and understandable, allowing for better public scrutiny.
Can AI systems make ethical decisions
AI systems currently cannot make ethical decisions on their own, despite their growing complexity. Here’s a breakdown of why;
- Lack of moral reasoning: AI works by identifying patterns and making predictions based on data. It can’t understand ethical principles, consider the nuances of a situation, or grasp the broader societal impact of its choices.
- Reliance on data: AI decisions are only as good as the data they’re trained on. Biased data can lead to biased decisions, even if the AI itself isn’t inherently biased.
- Focus on optimization: AI systems are typically designed to optimize for a specific outcome, like maximizing profit or minimizing risk. This narrow focus can lead to ethically questionable decisions if it doesn’t take into account broader human values.
However, AI can still be a valuable tool in ethical decision-making by;
- Identifying and mitigating bias: AI can analyze data to uncover potential biases in human decision-making processes.
- Providing additional information: AI can analyze vast amounts of data to present a more comprehensive picture of a situation, allowing for more informed ethical choices.
- Automating routine tasks: Freeing up human time for decisions that require ethical judgment and empathy.
How to ensure accountability in AI systems
Ensuring accountability in AI systems is crucial for building trust and mitigating risks. Here are some key approaches;
1. Understanding the AI Lifecycle
- Design: Clearly define the system’s objectives, potential biases, and how it aligns with ethical principles.
- Development: Document data sources, training methods, and human oversight mechanisms.
- Deployment: Monitor performance, and identify and address unintended consequences.
- Monitoring: Continuously assess fairness, bias, and societal impact. Be prepared to refine or retire the system if necessary.
2. Transparency and Explainability
- Algorithmic transparency: Strive to make AI decisions understandable, even if the inner workings are complex.
- Explainable AI techniques: Develop methods to explain how AI arrives at specific outputs.
3. Data Accountability
- Data provenance: Track the origin and usage of data throughout the AI lifecycle.
- Data bias detection: Identify and mitigate biases within the data used to train AI models.
- Data privacy protections: Ensure compliance with data privacy regulations and user consent.
4. Roles and Responsibilities
- AI developers: Responsible for designing, building, and testing AI systems to be fair, unbiased, and secure.
- Organizations deploying AI: Implement policies and procedures for responsible AI use, monitoring performance, and addressing issues.
- Regulatory bodies: Develop and enforce regulations that promote fairness, transparency, and accountability in AI.
5. Human Oversight and Control
- Maintain human oversight: Humans should make final decisions in critical situations and hold ultimate accountability for AI actions.
- Human-in-the-loop systems: Design AI systems that involve human input and judgment at key stages.
6. Building a Culture of AI Ethics
- Promote awareness and education: Educate developers, users, and the public about the potential risks and benefits of AI.
- Develop ethical guidelines: Establish clear ethical principles to guide the development and deployment of AI.
What ethical guidelines exist for the development of AI
Several ethical guidelines and principles have been proposed for the development and deployment of AI systems. Some of the most prominent ones include;
- Beneficence: AI systems should be designed to benefit individuals and society as a whole, maximizing the overall well-being of humanity.
- Non-maleficence: Developers should strive to minimize harm caused by AI systems. This includes avoiding biases, discrimination, and unintended negative consequences.
- Fairness and Equity: AI systems should be designed and implemented in a way that promotes fairness and avoids reinforcing or exacerbating existing inequalities and biases.
- Transparency: Developers should strive to make AI systems transparent and understandable to users and stakeholders, enabling them to comprehend how decisions are made and actions are taken.
- Accountability and Responsibility: Those involved in the development and deployment of AI systems should be accountable for their actions and decisions, and mechanisms for recourse and redress should be in place in case of errors or harm.
- Privacy and Data Protection: AI systems should respect individuals' privacy rights and handle personal data responsibly, adhering to relevant privacy laws and regulations.
- Security: AI systems need to be equipped with strong security measures to defend against unauthorized access and data breaches.
- Human Control and Autonomy: Humans should maintain control over AI systems, ensuring that they are used to augment human capabilities rather than replace human decision-making autonomy.
- Societal and Environmental Well-being: AI development should consider broader societal and environmental impacts, including factors such as job displacement, environmental sustainability, and the overall welfare of communities.
- Continual Assessment and Improvement: Developers should continuously assess the ethical implications of AI systems throughout their lifecycle and be prepared to make adjustments to address emerging issues or concerns.
Are there concerns about AI and job displacement
Yes, there are significant concerns about AI and job displacement. Here’s a breakdown of the issue;
- AI automation: AI is rapidly automating tasks previously done by humans, particularly repetitive and routine tasks. This displaces workers in certain sectors, like manufacturing or data entry.
- Impact on specific jobs: Jobs with a high degree of predictability and standardization are most at risk of being automated by AI. This could significantly impact sectors like transportation (e.g., self-driving trucks), customer service (e.g., chatbots), and even some aspects of healthcare diagnosis.
- Uncertain pace of job creation: While AI may create new jobs, it’s uncertain how quickly these new jobs will emerge and whether they will offset the jobs lost to automation. This could lead to a period of unemployment and economic disruption.
- Impact on specific demographics: Concerns exist that AI could disproportionately impact certain demographics, such as low-skilled workers or those without the training or education to transition to new jobs created by AI.
Here are some counterarguments to consider;
- History of job creation: Throughout history, technological advancements have often led to job displacement in some sectors, while creating new opportunities in others. The net effect has typically been an increase in overall job creation.
- AI as a complementary tool: AI may not replace workers entirely, but rather augment human capabilities, allowing people to focus on more creative and strategic tasks.
- Upskilling and retraining: By focusing on education and retraining programs, we can equip workers with the skills needed to thrive in the AI economy.
How does AI affect social justice issues
AI can impact social justice issues in both positive and negative ways. Here’s a breakdown of the key areas;
Negative impacts
- Bias and discrimination: AI algorithms trained on biased data can perpetuate or amplify existing societal biases. This can lead to unfair outcomes in areas like loan approvals, criminal justice (e.g., risk assessment tools), or facial recognition technology.
- Job displacement: AI automation threatens to replace jobs, particularly those involving routine tasks. This could exacerbate economic inequality if new jobs created by AI require different skillsets that leave some workers behind.
- Privacy concerns: AI systems often rely on vast amounts of personal data, raising concerns about surveillance and potential misuse of information. This can disproportionately impact marginalized communities already facing higher scrutiny.
- Lack of transparency and accountability: The complex nature of some AI algorithms can make it difficult to understand how they arrive at decisions. This lack of transparency can hinder accountability and make it challenging to address bias or errors.
Positive impacts
- Promoting fairness: AI tools can be used to analyze data and identify potential biases in existing systems, helping to create fairer processes.
- Empowering social justice movements: AI can be used to analyze social media data to track hate speech or identify areas of discrimination. It can also be used to automate administrative tasks in social justice organizations, freeing up human resources for more strategic work.
- Improving access to services: AI can be used to develop personalized education or healthcare programs, potentially improving access to essential services for underserved communities.
- Augmenting human decision-making: AI can analyze vast amounts of data to inform decision-making, potentially leading to more evidence-based and objective outcomes.
Moving forward
- Developing ethical guidelines: Clear ethical frameworks for AI development are crucial to mitigate risks and ensure social justice principles are considered.
- Promoting diversity and inclusion in AI: Encouraging diverse perspectives in AI development can help identify and address potential biases.
- Public awareness and education: Increasing public understanding of AI and its potential impacts is essential for promoting responsible development and use.
What are the ethical considerations in AI healthcare applications
AI in healthcare holds immense promise for improving diagnoses, treatment plans, and overall patient outcomes. However, alongside its benefits lie a number of ethical considerations that need to be addressed to ensure responsible and equitable use. Here’s a breakdown of some key areas;
1. Bias and Fairness
- Training data bias: AI algorithms trained on biased medical datasets can perpetuate discrimination against certain demographics in areas like disease diagnosis, treatment recommendations, or insurance eligibility.
- Algorithmic bias: Even unbiased data can lead to biased algorithms if they give more weight to certain factors that unfairly disadvantage certain groups. This could lead to misdiagnoses or unequal access to care.
2. Transparency and Explainability
- “Black box” algorithms: The complex nature of some AI healthcare systems can make it difficult to understand how they arrive at diagnoses or treatment suggestions. This lack of transparency can erode trust and make it hard to identify and address errors.
- Explainable AI (XAI): There’s a growing need for XAI techniques to make AI healthcare decisions more transparent, allowing doctors to understand the reasoning behind the AI’s outputs.
3. Patient Privacy and Data Security
- Highly sensitive data: Healthcare data is highly sensitive and vulnerable to misuse. Robust data security measures are crucial to prevent breaches and protect patient privacy.
- Informed consent: Patients need clear and comprehensive information on how their data is being used in AI applications, and they should have the right to opt-out if they wish.
4. Accountability and Liability
- Who’s responsible?: When an AI system makes a mistake in healthcare, it’s unclear who should be held accountable – the developers, the healthcare providers, or the AI system itself. Clear legal frameworks are needed to address liability issues.
5. Human Oversight and Control
- AI as a tool, not a replacement: AI should be seen as a tool to augment human expertise, not replace it. Ultimately, medical decisions should involve human judgment and compassion alongside AI insights.
6. Access and Equity
- Socioeconomic disparities: Unequal access to AI-powered healthcare services could exacerbate existing disparities. Efforts are needed to ensure everyone benefits from AI advancements, regardless of background.
Are there regulations governing the ethical use of AI
There are currently no universally accepted regulations governing the ethical use of AI. However, there are several moving parts in this area, including;
- International principles: The United Nations Educational, Scientific and Cultural Organization (UNESCO) has developed a comprehensive framework based on human rights principles. Their “Recommendation on the Ethics of Artificial Intelligence” https://www.unesco.org/en/artificial-intelligence/recommendation-ethics outlines principles like fairness, transparency, accountability, and human oversight.
- Regional and National Efforts: The European Union (EU) and many national governments are developing their own ethical guidelines for AI. These guidelines often focus on areas like transparency, accountability, human-centricity, and respect for privacy. For instance, Australia has its own AI Ethics Framework https://www.industry.gov.au/publications/australias-artificial-intelligence-ethics-framework.
- Industry Standards: Some industry organizations are developing standards for ethical AI development. The Institute of Electrical and Electronics Engineers (IEEE) has created a standard on ethical considerations in artificial intelligence and autonomous systems https://standards.ieee.org/wp-content/uploads/import/documents/other/ethical-considerations-ai-as-29mar2018.pdf.
Challenges in Regulation
- Rapid pace of AI development: Regulations struggle to keep pace with the rapid advancement of AI technology.
- Balancing innovation and control: Striking a balance between encouraging innovation and implementing effective safeguards is crucial.
- Global vs. national regulations: AI development and use is a global issue, so ensuring internationally consistent regulations is a challenge.
What are the ethical dilemmas of autonomous vehicles
Autonomous vehicles (AVs) present a future filled with convenience and potentially less traffic congestion. However, along with the excitement come significant ethical dilemmas that need to be addressed before widespread adoption. Here are some key areas of concern;
1. The Trolley Problem on Autopilot
Imagine an unavoidable crash scenario: the AV swerves to avoid a pedestrian but risks hitting a group of people on the sidewalk. Here’s the ethical dilemma;
- The Programmer’s Choice: Who should the car be programmed to prioritize in such situations? Utilitarian ethics might dictate minimizing total casualties, but this assigns an impossible moral burden to programmers.
- No Easy Answers: There’s no universally accepted solution to these “trolley problem” scenarios, and placing the responsibility on the AV itself raises further ethical concerns.
2. Transparency and Accountability
- “Black Box” Decisions: The inner workings of some AV algorithms might be complex and opaque. In an accident, it could be difficult to determine who’s accountable - the manufacturer, the programmer, or the car itself?
- The Need for Explainability: There’s a growing need for more transparent and explainable AV decision-making processes. This would allow for better scrutiny and accountability in case of accidents or malfunctions.
3. Data Privacy and Security
- Vast Amount of Data: AVs collect a massive amount of data from sensors and cameras, raising privacy concerns. How will this data be stored, used, and protected from hacking?
- Mitigating Privacy Risks: Robust data security measures and clear regulations on data usage are crucial to ensure individual privacy and prevent misuse of this sensitive information.
4. Ethical Hacking and Security Threats
- Vulnerability to Attacks: AVs are complex computer systems, and like any computer system, they are vulnerable to hacking. A hacked AV could cause serious accidents or privacy breaches.
- Prioritizing Cybersecurity: Cybersecurity needs to be a top priority throughout the design, development, and deployment of AVs.
5. The Impact on Jobs and Inequality
- Job Displacement: Widespread AV adoption could lead to job losses for taxi drivers, truck drivers, and others in the transportation sector.
- Ensuring Equitable Access: The transition to AVs needs to be managed carefully to mitigate job displacement and ensure that the benefits of this technology are equitably distributed across society.
How does AI impact environmental sustainability
AI has the potential to be a powerful tool for environmental sustainability, offering solutions and insights across various areas. Here’s a breakdown of the positive impacts;
- Optimizing Resource Management: AI can analyze data on energy use, water consumption, and resource allocation to identify inefficiencies and opportunities for optimization. This can lead to significant reductions in energy use in buildings and industries, smarter irrigation practices in agriculture, and better waste management strategies.
- Monitoring and Conservation Efforts: AI-powered tools can be used to monitor environmental changes, track deforestation, and detect illegal activities like poaching. Machine learning algorithms can analyze satellite imagery and sensor data to identify trends and potential environmental threats.
- Developing Renewable Energy Sources: AI can assist in designing more efficient solar panels, wind turbines, and energy storage systems, accelerating the transition to renewable energy sources. It can also optimize the placement of renewable energy infrastructure for maximum efficiency.
- Precision Agriculture: AI can help farmers apply fertilizers, pesticides, and water more precisely, reducing waste and environmental impact. It can also be used to monitor crop health and predict yields, allowing for more sustainable agricultural practices.
- Climate Change Mitigation: AI can be used to model climate change scenarios and design strategies to mitigate its effects. AI can analyze vast datasets to understand complex weather patterns and predict extreme weather events.
However, it’s important to acknowledge the potential drawbacks of AI for the environment;
- Increased Energy Consumption: Training and running complex AI models requires significant computing power, which can lead to a higher carbon footprint if not powered by renewable energy sources.
- Data Center Impact: The data centers that house AI systems require a lot of energy for cooling and operation. Sustainable data center design and practices are crucial to minimize the environmental impact of AI.
- Reliance on Rare Earth Metals: Some AI hardware components rely on rare earth metals, mining of which can have negative environmental consequences. Finding sustainable alternatives for these materials is important.
Overall, AI presents a significant opportunity to advance environmental sustainability. By acknowledging the challenges and implementing responsible practices, we can ensure that AI is used as a force for good in protecting our planet. Here are some additional points to consider;
- Importance of Collaboration: Collaboration between AI researchers, environmental scientists, and policymakers is crucial to maximize the positive environmental impact of AI.
- Focus on Sustainability in AI Development: The development of AI systems themselves needs to be sustainable, considering factors like energy efficiency and responsible data management.
- Public Awareness and Education: Raising public awareness about the potential of AI for environmental sustainability can encourage broader support for responsible AI development and deployment.
Can AI be used ethically in military applications
The use of AI in military applications raises a complex ethical debate. Here’s an analysis of the possible advantages and disadvantages to take into account;
Potential Benefits
- Improved Targeting and Reduced Civilian Casualties: In theory, AI could improve targeting precision, potentially reducing civilian casualties in armed conflicts. This is because AI can analyze vast amounts of data and make split-second decisions faster than humans, potentially filtering out unintended targets.
- Enhanced Situational Awareness: AI systems can analyze data from multiple sensors (drones, satellites) to provide a more comprehensive picture of the battlefield, potentially reducing friendly fire incidents and improving overall mission effectiveness.
- Reduced Risk to Soldiers: Autonomous systems like bomb disposal robots or sentry drones could reduce the risk of human casualties in high-risk situations.
- Logistics and Resource Management: AI can be used for logistics optimization, predictive maintenance of equipment, and streamlining military operations, potentially leading to more efficient use of resources.
Ethical Concerns and Drawbacks
- Autonomous Weaponry: The development of fully autonomous weapons systems that can select and engage targets without human intervention raises serious ethical concerns. Who is responsibile if an autonomous weapon makes a mistake? These systems could also destabilize international relations and lower the threshold for armed conflict.
- Bias and Discrimination in Targeting: AI algorithms trained on biased data could perpetuate discrimination in targeting decisions. Ensuring fairness and unbiased decision-making in military AI is crucial.
- Lack of Human Judgment and Accountability: Complex ethical decisions about the use of force should ultimately remain with humans. Over-reliance on AI for military decision-making removes human judgment from the equation and makes it difficult to assign accountability.
- The Risk of Arms Race: A global arms race in AI-powered weaponry could lead to unpredictable and potentially catastrophic consequences. International cooperation and arms control measures are essential.
- Psychological Impact and Dehumanization: The widespread use of autonomous weapons could distance soldiers from the act of killing, potentially leading to psychological harm and a dehumanization of warfare.
The question of whether AI can be used ethically in the military doesn’t have a simple answer. It depends on the specific application, the level of human oversight, and the existence of robust international regulations governing its development and use.
Here are some additional points to consider;
- Importance of International Dialogue: Open and transparent dialogue between nations regarding ethical AI development and use in the military is essential for mitigating risks and building trust.
- Focus on Human-AI Teaming: The ideal scenario might involve human-AI teaming, where AI augments human capabilities but ultimate decision-making authority rests with humans.
- Focus on Defensive Applications: If AI is to be used militarily, focusing on defensive applications like missile defense systems might be more ethically acceptable than offensive capabilities.
What are the ethical implications of AI in surveillance
AI-powered surveillance systems are becoming increasingly sophisticated, raising a multitude of ethical concerns. Here’s a breakdown of the key issues;
- Privacy Violations: Extensive surveillance using facial recognition, movement tracking, and data collection from cameras and sensors can have a chilling effect on privacy. It can create a sense of being constantly monitored, stifling free expression and dissent.
- Bias and Discrimination: AI algorithms used in surveillance can be biased, leading to unfair targeting and discrimination against certain demographics. For example, facial recognition systems have been shown to have higher error rates for people of color.
- Mass Surveillance and Chilling Effect: The possibility of constant, large-scale surveillance can lead to a self-censorship phenomenon, where people avoid expressing certain views or engaging in certain activities for fear of being monitored.
- Lack of Transparency and Accountability: The inner workings of some AI surveillance systems are complex and opaque. This lack of transparency makes it difficult to hold authorities accountable for misuse or errors.
- Potential for Abuse: AI-powered surveillance systems in the wrong hands could be used to repress dissent, crack down on minorities, or curtail civil liberties.
Here are some countervailing arguments in favor of AI surveillance;
- Crime Prevention and Public Safety: Proponents argue that AI surveillance can be a valuable tool for law enforcement, deterring crime and improving public safety.
- Finding Missing Persons and Solving Crimes: AI can assist in analyzing vast amounts of data to identify patterns and find missing persons or solve crimes more quickly.
Moving forward, it’s crucial to find a balance
- Clear Regulations and Oversight: Robust regulations governing data collection, storage, and use for surveillance are essential. Independent oversight mechanisms are needed to ensure accountability.
- Transparency and Public Education: There needs to be greater transparency about how AI surveillance works and clear guidelines on its use. Public education can foster informed debate about the trade-offs between security and privacy.
- Focus on Targeted Surveillance: Surveillance efforts should be targeted and based on reasonable suspicion, not mass indiscriminate monitoring.
- Alternatives to AI Surveillance: Exploring alternative methods for crime prevention and public safety should be prioritized, such as community policing and addressing the root causes of crime.
How should AI systems handle sensitive personal data ethically
Here are some key principles for AI systems to handle sensitive personal data ethically;
1. Transparency and User Control
- Clear Communication: Be transparent about what data is collected, how it’s used, and who has access to it. Provide users with clear and understandable explanations of AI processes.
- Data Minimization: Collect only the data essential for the AI system’s function. Avoid collecting unnecessary personal data.
- User Consent: Obtain clear and informed user consent for the collection, use, and storage of their personal data. Allow users to easily withdraw consent at any time.
- Access and Control: Give users the right to access their data, request corrections, and have their data deleted under certain circumstances (e.g., right to be forgotten).
2. Security and Privacy
- Robust Security Measures: Implement strong security measures to protect sensitive data from unauthorized access, use, disclosure, disruption, modification, or destruction.
- Data Anonymization and Pseudonymization: Where possible, anonymize or pseudonymize data to minimize privacy risks. This reduces the possibility of re-identification of individuals.
- Regular Security Audits: Conduct regular security audits to identify and address vulnerabilities in data storage and processing systems.
3. Fairness and Non-discrimination
- Data Bias Detection: Proactively identify and mitigate biases within the data used to train AI models. Biased data can lead to discriminatory outcomes.
- Fairness Testing: Test AI systems for potential biases to ensure they don’t discriminate against certain demographics based on factors like race, gender, or religion.
- Explainable AI (XAI): Develop AI systems that are explainable, allowing users to understand how their data is being used and why the AI arrives at certain decisions.
4. Accountability and Legal Compliance
- Clear Lines of Responsibility: Establish clear lines of accountability for data governance and ensure compliance with relevant data privacy regulations (e.g., GDPR, CCPA).
- Data Protection Impact Assessments: Conduct Data Protection Impact Assessments (DPIAs) to evaluate the potential risks associated with processing personal data.
- Adherence to Ethical Principles: Align AI development and use with established ethical principles for AI, such as those outlined by the OECD or UNESCO.
5. Algorithmic Governance
- Human Oversight: Maintain human oversight throughout the AI lifecycle, from development and deployment to monitoring and evaluation.
- Human-in-the-Loop Systems: Design AI systems that involve human review and intervention at critical stages, especially when sensitive data is involved.
- Continuous Improvement: Continuously monitor and improve AI systems to ensure they remain fair, accountable, and aligned with ethical principles.